How to learn machine learning and improve your health at the same time
Here’s a new rule: If your model is moving, you’re moving.
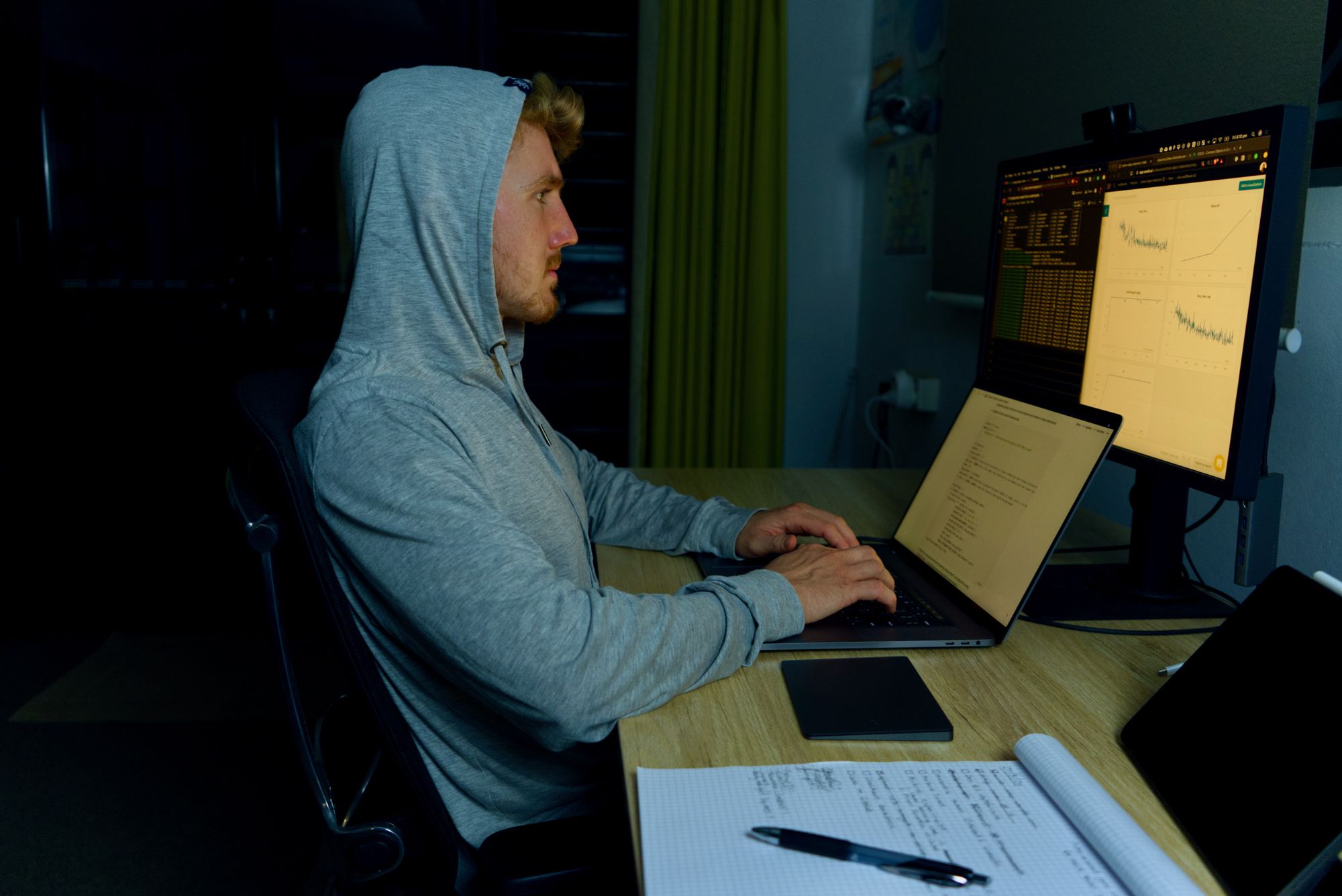
Now’s a good time to ask yourself two questions.
- What’s not going to change?
- What’s going to change?
The time after which nothing was the same
That’s where the best stories start. Or at least they contain an arc which takes a character through a series of events after which nothing is the same.
Let’s pretend this character is you (and me, because I’m thinking about these things too).
When things go back to normal, what’s going to look different to you?
First, it must be understood, there is no universal definition of normal. You’ve got your story, I’ve got mine. And you know what?
The beautiful thing about being the main character in your story is you get to create it. You get to write about what changes and what doesn't.
Perhaps you’ve realised half the activities you do aren’t required. Or half the crap you own doesn’t bring you joy.
A formula for learning machine learning (or anything)
I published a video called Don’t learn machine learning at the end of last month. A clickbaity title for an underlying message.
Avoid being a donkey.
How does one avoid being a donkey?
By recognizing the donkey problem when it appears and acting accordingly.
The donkey problem comes from a story where a donkey is stuck between a bucket of water and a pile of food. It can’t decide if it’s hungry or thirsty and so it glances at the water, then at the food, then back at the water, food, water, food, water.
Eventually, unable to decide on anything, the donkey dies of hunger and thirst.
Perhaps you’ve experienced this problem before. Being stuck between two (or more) things and being so absorbed in the consideration of each that none eventuate.
Now.
There are plenty of ways to look at this. Perhaps, procrastinating on a decision can be helpful.
The longer you wait, the smarter you become, the better decision you make.
Or the procrastination itself is a sign none the options are viable and you should choose none. You know how the saying goes, if you can’t decide, the answer is no.
Let’s tie this back to learning machine learning.
The most consistent question I get asked is, “how do I learn machine learning?”.
Often it’ll come along with things like.
“I’ve got no math experience, can I still learn it?”
“I don’t have a background in statistics or neuroscience, what should I do?”
To which my first reaction is “if everyone needed background in something before they started, no one would start anything.”
I don’t say this, of course, I’m more polite in my responses but I can be more direct in this article.
The donkey problem here is clear. Someone has an interest in learning a new topic but is held back by considering the infinite paths which reside in front of them.
The fix?
If none of the existing paths in front of you work, you must create your own.
Here’s one sure-fire way.
- Use online courses and materials (you’re smart enough to find these) to build a foundation of knowledge. Go for skills, not certificates.
- Turn your foundation of knowledge into specific knowledge (knowledge which can’t be taught) through creating projects of your own.
Questions.
Which resources are the best?
Many of them are more than enough to get started. Read the reviews. Try one, see if it sparks your curiosity enough to keep going.
What if there’s something better out there?
Donkey problem. Avoid. You’ll figure this out by trying something, seeing if it works for you and moving on if it doesn’t. Remember, you’re smarter doing than you are thinking.
I’ve done some introductory courses, what projects should I work on?
Your own.
How?
Design something which might not work.
Build a toy to impress your customers, your fans, yourself. Don’t worry about making something to impress the competition or your peers.
What if my efforts are wasted?
99% of all effort is wasted. But if you never dedicate any, you’ll never get the chance to strike the 1% which isn’t.
Notice how what we’ve gone through can be applied to learning almost anything.
The most important takeaways being instead of focusing on what’s right at any given moment (impossible to predict), you’re concentrating on the trend.
You’re building the habit of learning (using courses as a foundation) along with the habit of creating (building your own projects).
After a while, you’ll find one implies the other. Soon enough, your creations will fuel your learning and your learning will fuel your creations.
The tools and resources you use to learn something will change over time but how, building a habit of learning, building a habit of experimenting won’t.
Improve your health at the same time as learning machine learning
I promised health would be included here.
Health isn’t going to change.
Lose your health and what do you have?
If you’re learning machine learning, I’m going to give you a new rule.
Every time your model is moving, your body is moving.
Imagine you’re training a neural network or doing a grid search with a random forest. Model moving. Body moving.
Perhaps you go for a walk, do some pushups or stretch those tight hips from prolonged sitting. After which, you come back and check the results. And the good news is despite your loss function not decreasing at all (you forgot to add optimizer.step()
), your body feels great.
Why?
A lack of movement leads to a clogged body. When your body is clogged, your mind is clogged. And you don’t want that.
Again, the parallel can be drawn back to learning a new topic.
Of any given topic (especially machine learning) the resources to get started can be vast. Thus the high potential for a donkey problem.
Same with health, there are many dogmas of eating, moving but none are as important as the trend.
Let me be clear.
The habit of movement is more important than the kind of movement.
The habit of learning and using what you’ve learned to create is more than the resources you use to learn.
If you want to improve your health, your knowledge, your ability to break down problems and build solutions, the best way is in the form of small actions compounding over time.
Create your own feedback loop
Okay.
You might be thinking, “Daniel, I’m stuck at a donkey problem, what can I do?”.
Aside from what we’ve talked about, here are a couple of steps you can take.
Rate things on a scale
Your health, your learning, your creative outlets, your relationships, other things which are important to you. How do they stack up from 1 to 5? The lowest ones should be addressed first. After you’ve finished reading this article, write them down.
30 days
After you’ve got your ratings, devise 1–3 steps you could take for the lowest one(s) over the next 30 days. Less is more here. Start with the smallest possible action. It might be spending 10 minutes walking or reading per day. Or removing the 15 tabs you have bookmarked and sticking with the one which sparks your curiosity most.
The follow-up
A neglected point in any interaction is the follow-up. Don’t underestimate it. At the end of the 30 days redo the ratings and ask yourself:
- What have I removed? (remember, if you’re reading this, it’s likely your life will improve via subtraction, not addition).
- What have I learned?
- What have I created?
- How have I moved?
- What’s staying the same?
- What’s changing for the next 30-days?
This activity doesn’t have to take more than an hour. But what you’re doing is creating your own feedback loop. Completely self-driven.
Because.
As you know, no one cares about you as much as you. Whether it’s learning something new or taking care of your health, it’s on you.
Need inspiration?
I made a video of me following the new rule: model moving, body moving. Come and follow along.
This article originally aired as the April 2020 issue of Eat, Move, Learn, Make, a newsletter I send out once a month or so for hungry, active, curious creators. You might be one. For more works like this, be sure to sign up.