Single Machine Learning Engineer Takes Laptop on a Date for Valentine’s Day
A story of love and loss functions.
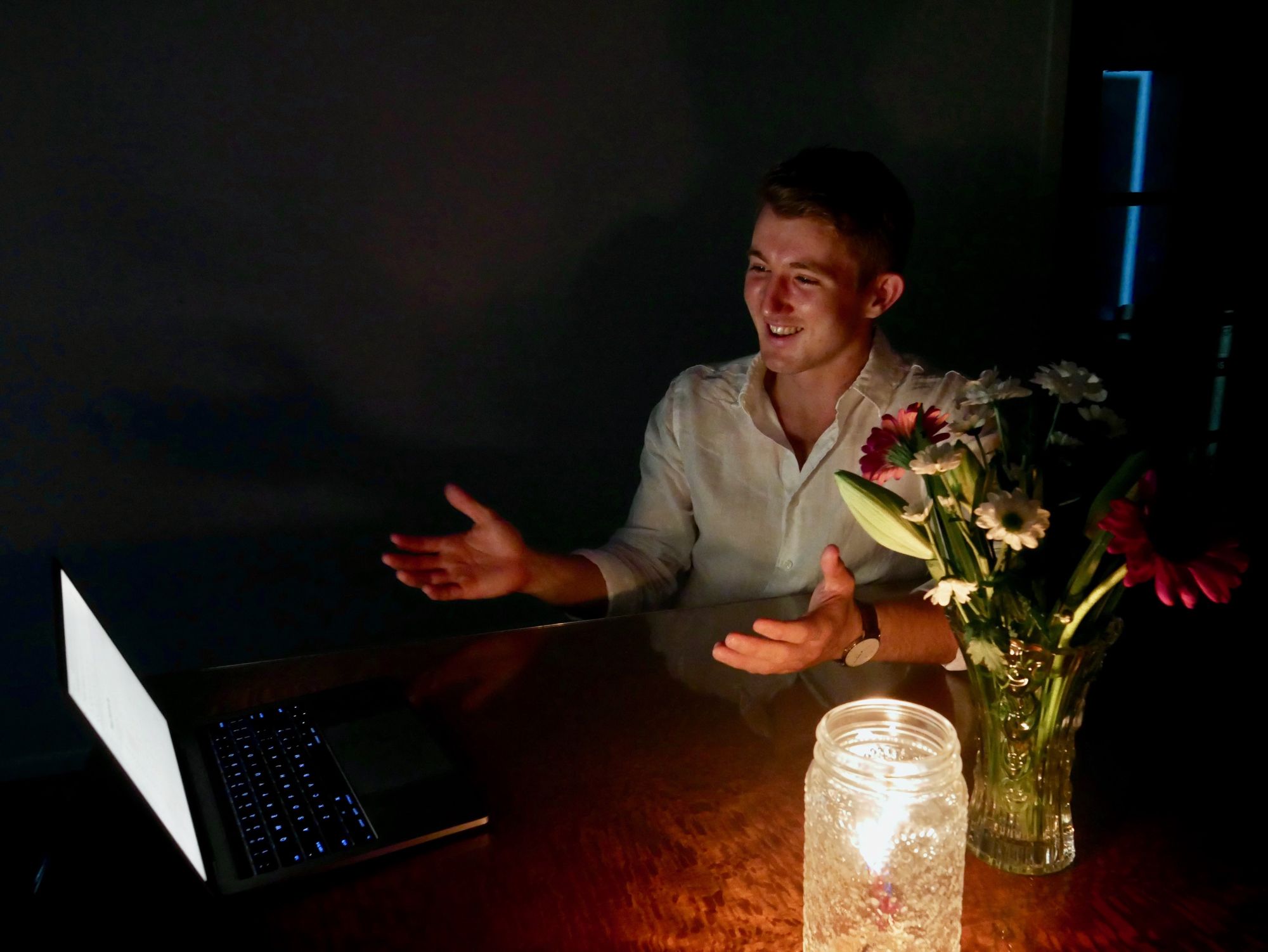
A story of love and loss functions
It was happening. Date night. While everyone was out spending big, I was going to be the outlier and cook at home. I’ve optimised plenty of cost functions before. Tonight would be no different.
I asked a friend for help. I had no idea what to cook.
You’d think after working so close together for the past 2–3 years I’d know a thing or two about my date.
Thai green chicken curry it was.
Food was like data I thought. You get a bunch of things together, combine them in some way and get something out at the other end. Something like that anyway.
I ordered 1kg worth of chicken and split it up into a training, validation and test sets. I’d preprocessed all the other ingredients a day before.
700g worth of chicken seemed like a lot for two people. But my friend sent me some good parameters to work with. After applying dropout, things seemed to normalise.
The recipe had the perfect learning rate. I even added in some of my own parallelisation by getting two pans on the go. Meat in one, vegetables in the other. I’d ensemble them together when they were ready.
“How’s this taste?” I asked my brother.
“10/10.”
Perfect accuracy. I was sceptical. Maybe I was overfitting on the training set. Accuracy wasn’t a good measure for cooking anyway. There was still 150g of chicken for validation.
“What about this?” I asked the same brother.
“8/10, the first one was better.”
“What do you think?” I asked my other brother.
“9/10, I’m a fan.”
I’d set up cross-validation. Ideally, I’d like to do more than 2-folds but it was too late. Dinner was in a few hours. Reinitialising the kitchen would take far too long.
The real test was about to begin. No instructions this time. If I hadn’t learned anything with the previous 850g of chicken, I was screwed.
What if the recipe didn’t hold together when it came time for the demonstration?
It was like going to show a client your work. You’d spent the last 6 weeks curating the most upvoted Stack Overflow Pandas functions together into an elegant looking pipeline only to have it fall over.
“I’m not sure what happened, it was working earlier on my end.”
Or worse. Someone forgot the dongle. The dongle. Always the dongle.
But you see that one was of the reasons I asked her to dinner. The uniqueness. She needed a dongle but didn’t need one at the same time.
She was shy, but there was this confidence about her. Those curves, the sparkle of the screen.
The table was set. Candles, flowers. We sat down. The conversation was one way as usual. I didn’t mind. It gave me time to air out my thoughts. No judgements. Love is the absence of judgement.
I started thinking. Maybe I was getting too deep too soon. What if I was adding all these layers to our relationship but all she wanted was something straight up and down. Something linear.
“Are you hungry?”
No response.
Maybe I said something wrong.
I went over and started cooking. It was show time. Cooking at home was the right choice. It meant I could run everything locally. In the same environment I’d practised on the training and validation set.
It was the same. Chicken in one pan. Vegetables in another. If it was anything like the validation set, it would take about 3-minutes to converge.
The sauce smelled amazing. Even better than before. Maybe the perfect accuracy on the training set wasn’t too far off.
I served up two bowls and sat down.
“This is Thai green chicken curry.”
No response.
I started eating. It tasted good. Really good.
What had I done wrong I wondered. I’d been through all the ingredients, got the inputs of others, checked my metrics.
Then it hit me. I’d been working with the wrong data the whole time. I’d spent all this time trying to provide an answer when I should’ve been asking more questions.
She didn’t want food. She didn’t need food. She was a laptop. Mine was up there but laptops don’t eat even the best Thai green curry.
The one thing she needed, I couldn’t provide.
I had a hunch but something blinded me. I was already in deep. It’s hard to step back when you’re already 15 layers in.
I plugged in the charger and she lit up like a Christmas tree. Mr Charger could provide.
“We can still be friends.”
I waited to hear it but it never came.
I went upstairs and cleared the table. Blew the candles out, put the extra bowl in the fridge.
When I came back downstairs they were still together.
I wasn’t silly. I knew there was a high probability they’d stay together. It hurt. It was a kind of loss to which I had no prior.
I told my friend the next day.
He took his hand off his chin and started talking.
“Look at this way, at least next time you can take more of a Bayesian approach.”